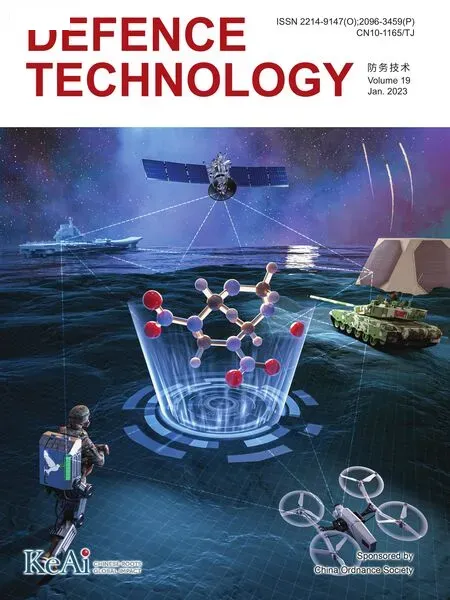
2023年1期
刊物介紹
《防務技術》(ISSN 1673-002X) 是由中國兵工學會主辦的科技類綜合性學術期刊,創(chuàng)刊于2005年,原名《兵工學報》英文刊,2013年起正式更名為《防務技術》,現(xiàn)為季刊。本刊發(fā)表基礎理論、應用科學和工程技術領域高水平原創(chuàng)性學術論文,包括理論研究、數(shù)值模擬和實驗研究類文章。其宗旨是通過這個平臺,報道和反映中國和國際防務技術領域的最新學術研究成果,主辦單位力爭在廣大科技工作者的支持下,用較短的時間,將其打造成為國際知名刊物。本刊專業(yè)范圍包括:裝甲、彈道、信息、計算機、材料、航空航天、力學等與防務技術相關的各個領域。
Defence Technology
- Experimental and numerical analysis on suitability of S-Glass-Carbon fiber reinforced polymer composites for submarine hull
- Damage response of conventionally reinforced two-way spanning concrete slab under eccentric impacting drop weight loading
- Analysis and design for the comprehensive ballistic and blast resistance of polyurea-coated steel plate
- Positive effects of PVP in MIC: Preparation and characterization of Al-Core heterojunction fibers
- Flight parameter calculation method of multi-projectiles using temporal and spatial information constraint
- Numerical simulation of drop weight impact sensitivity evaluation criteria for pressed PBXs
- Blast wave characteristics of multi-layer composite charge:Theoretical analysis,numerical simulation,and experimental validation
- Relationships between distribution characteristics of ceramic fragments and anti-penetration performance of ceramic composite bulletproof insert plates
- Dynamic mechanical characteristics of NdFeB in electromagnetic brake
- Sensitivity analysis of spacecraft in micrometeoroids and orbital debris environment based on panel method
- Quasi-static and low-velocity impact mechanical behaviors of entangled porous metallic wire material under different temperatures
- A small-spot deformation camouflage design algorithm based on background texture matching
- A review on the high energy oxidizer ammonium dinitramide:Its synthesis,thermal decomposition,hygroscopicity,and application in energetic materials
- Deep learning-based LPI radar signals analysis and identification using a Nyquist Folding Receiver architecture
- Task assignment in ground-to-air confrontation based on multiagent deep reinforcement learning